

Skills for Prosperity Kenya is a programme funded to strengthen existing digital (online, blended) education capacity for selected Public Higher Education Institutions (HEIs).
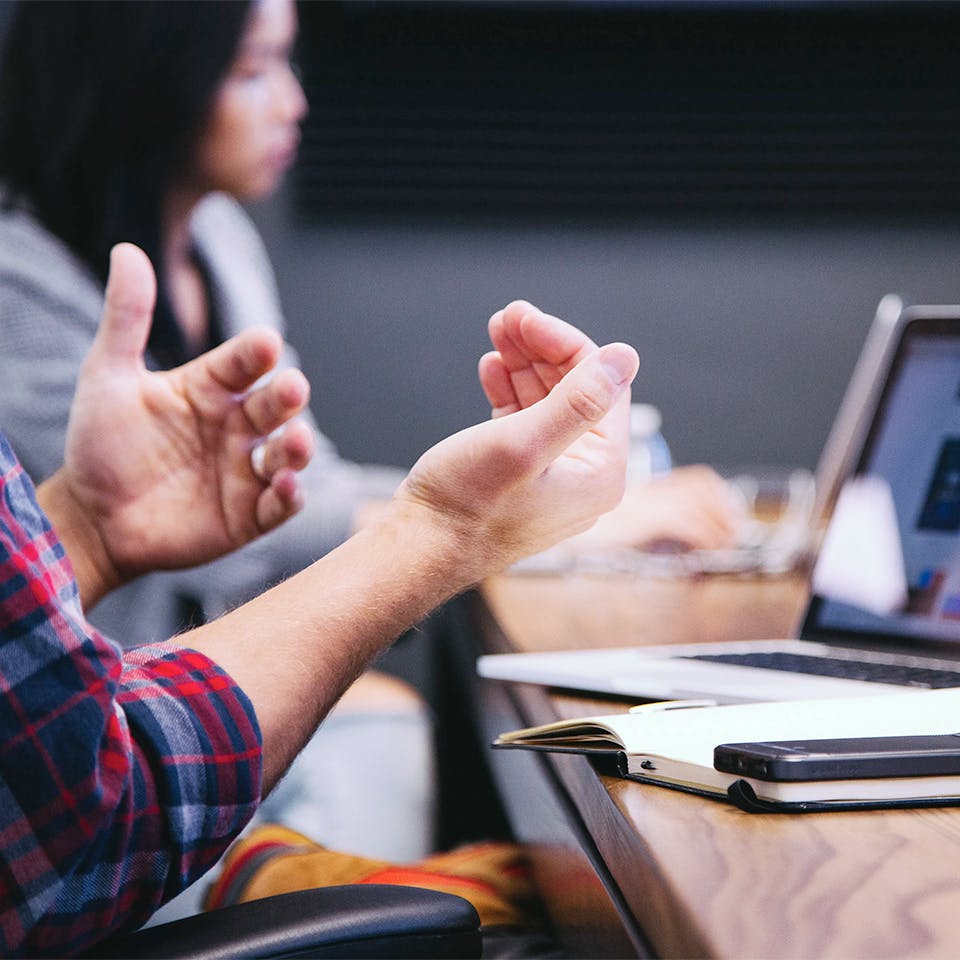

RESEARCH • EDITORIAL
The EU-funded ENCORE+ project conference took place during 30 November - 5 December, providing an opportunity for researchers to share findings, evidence and impact related to the use of open educational resources in education and business.
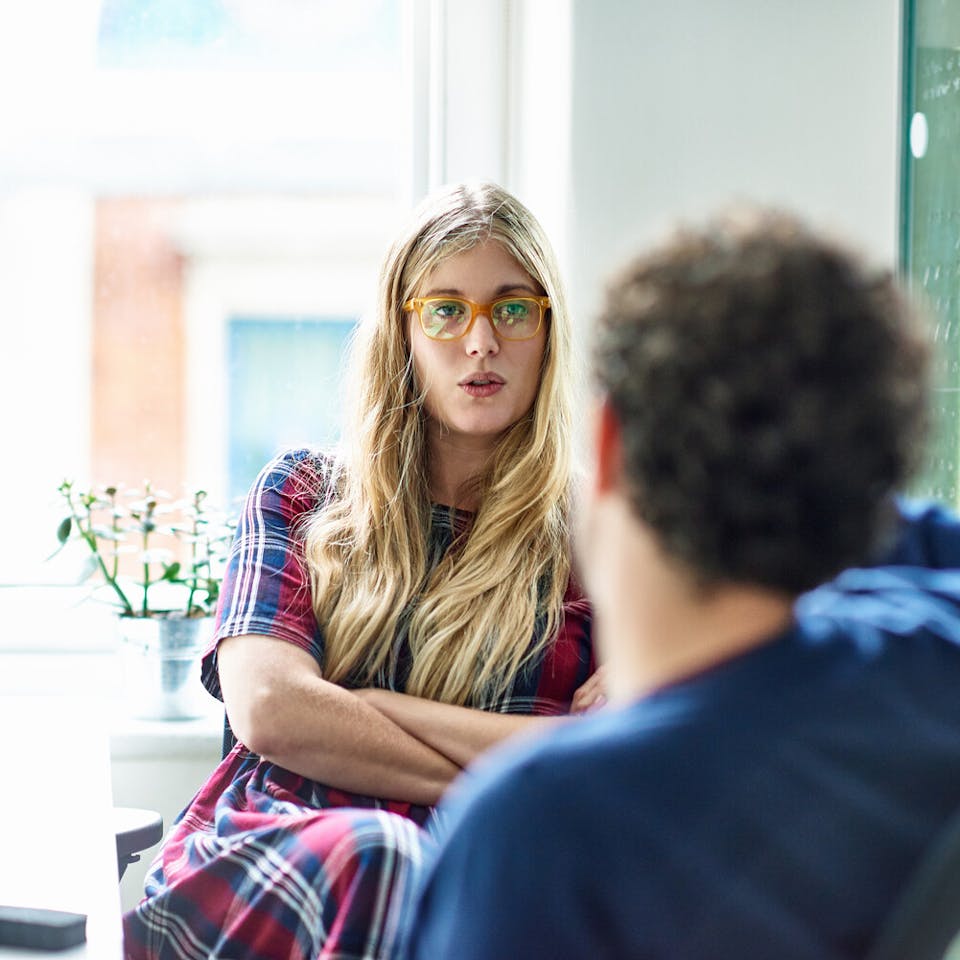

RESEARCH • EDITORIAL
A study conducted by researchers at The Open University’s Institute of Educational Technology and University College London has explored how disruption to studies can affect the mental health and wellbeing PhD students, with implications for research and university policy.
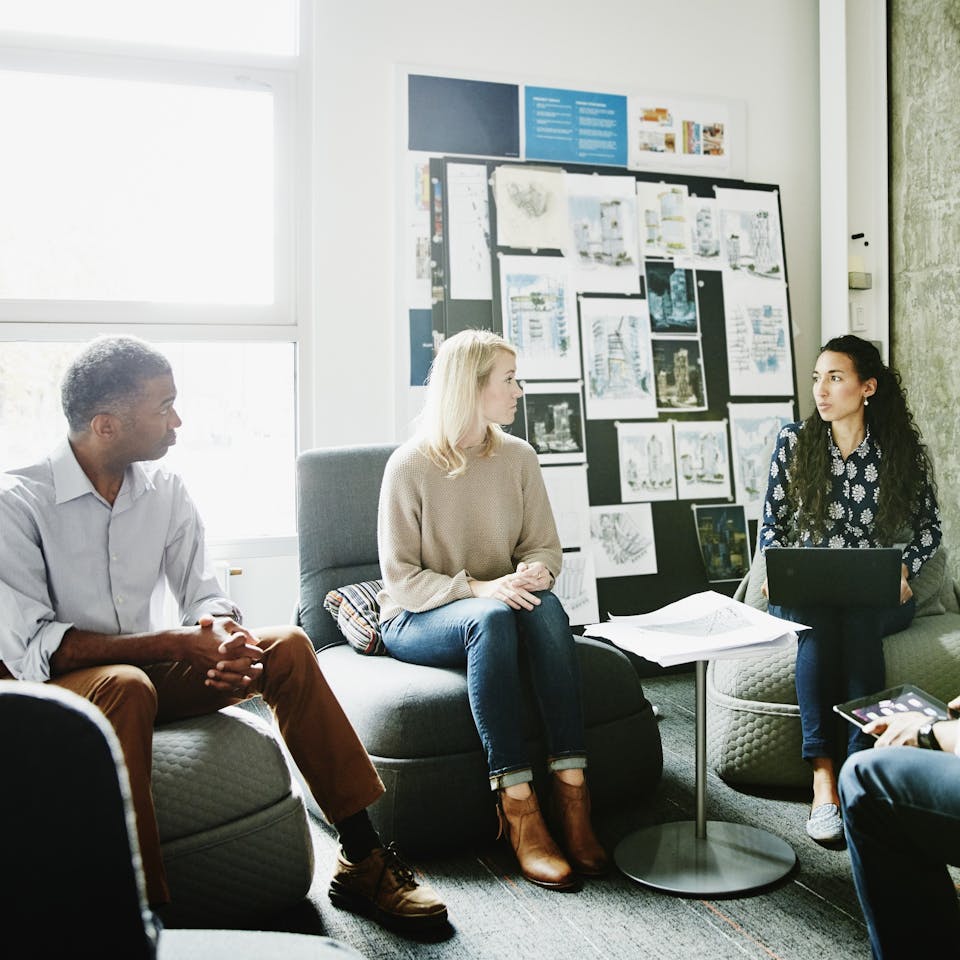

AN EDTECH FOUNDRY IN IET • EDITORIAL
Earlier this year The Open University (OU) invited EdTech startups to apply to join the IET EdTech Foundry, an accelerator established to speed up the scaling-out of research and innovation through knowledge exchange, across two phases of staged development.

RESEARCH • EDITORIAL
Dr Sewani attended The Open University during November-December 2023 to progress his research in open education on a prestigious Charles Wallace British Council Fellowship, managed by the British Council in Pakistan.
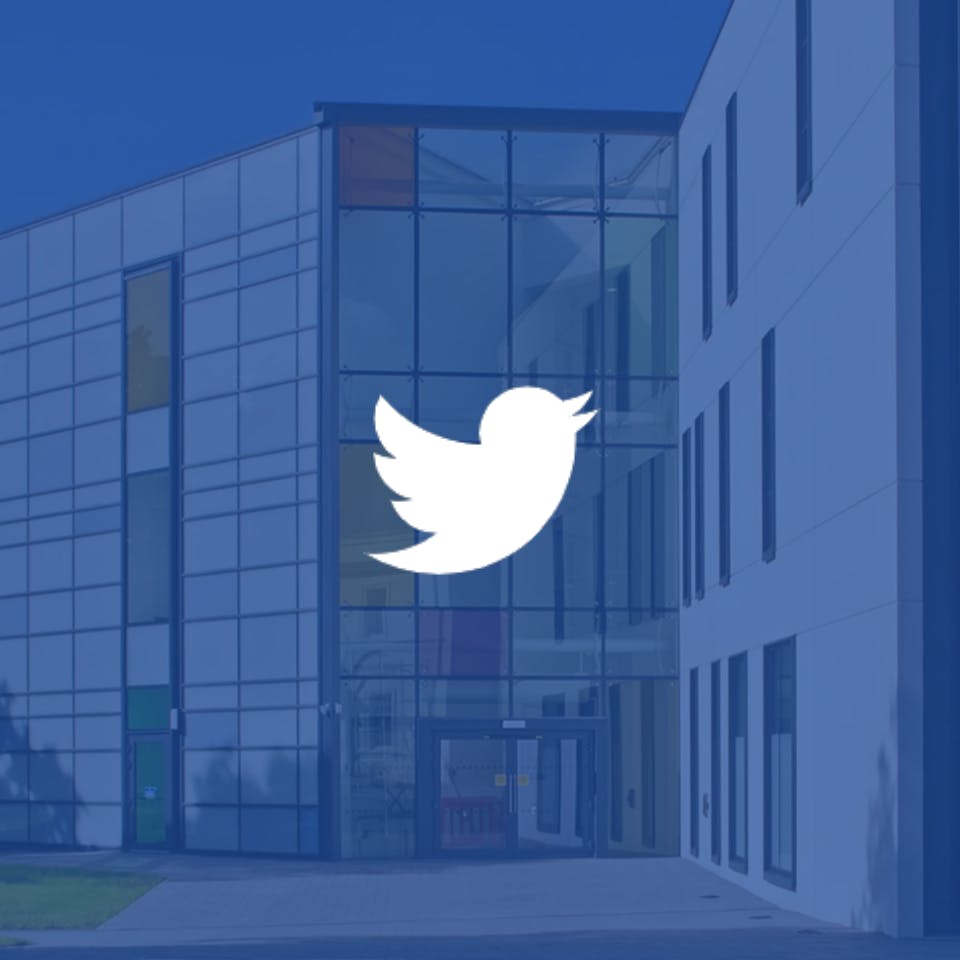

Tweet by @IETatOU •
The cut-off date for applications to study The Open University's Masters in Online Teaching, fully-funded with a Commonwealth Distance Learning Scholarship is 28 March. This Scholarship is open to citizens from developing Commonwealth countries. Find out more here.

STUDY WITH US • EDITORIAL
The globally available MA in Online Teaching offers a unique opportunity to study the theory and practice of online and blended teaching through a tailored combination of modules and professional development short courses delivered by The Open University (OU).

RESEARCH • EDITORIAL
OU research into Open Educational Resources has been awarded £546,759 for a fourth phase of funding by The William Flora and Hewlett Foundation.
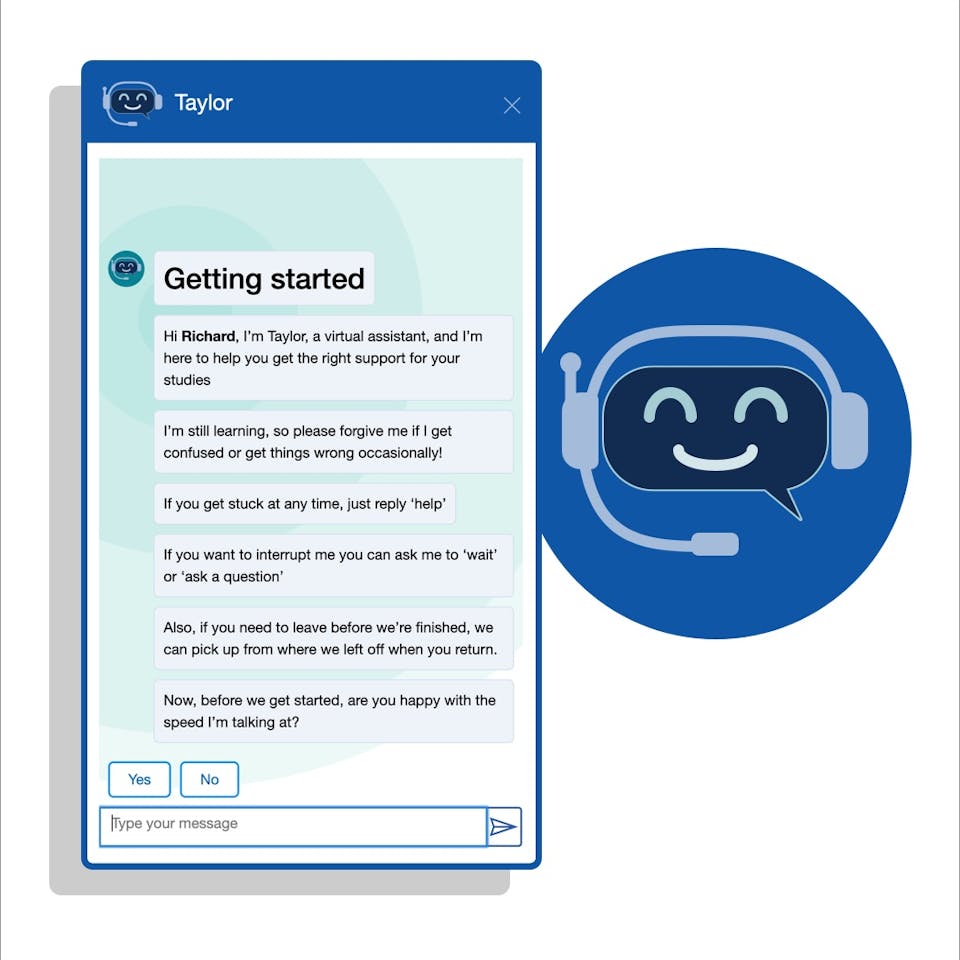

RESEARCH • EDITORIAL
Educational technologists at The Open University (OU) have developed a virtual assistant chatbot with artificial intelligence technology to support students to disclose disabilities and access information with ease.

RESEARCH • EDITORIAL
An interactive dashboard at The Open University (OU) is using machine learning methods to provide tutors (Associate Lecturers) with greater visibility of student engagement and experience.

INNOVATING PEDAGOGY • EDITORIAL
‘Metaverse’, 'artificial intelligence' and ‘Entrepreneurial education’ feature among the latest innovations for the future of education in the 11th edition of the Innovating Pedagogy Report.

RESEARCH • EDITORIAL
We are pleased to congratulate IET’s Dr Saraswati Dawadi and the OU’s Dr Margaret Ebubedike who have been voted the winner of The Open University’s People’s Choice Award.

RESEARCH • EDITORIAL
This month, Anne Adams, Professor of Engaged Practice and Research at the OU’s Institute of Educational Technology (IET), delivered her inaugural lecture on 'Why learners, politicians, practitioners and users are not the enemy and how to listen to them!'. Watch the full lecture below.
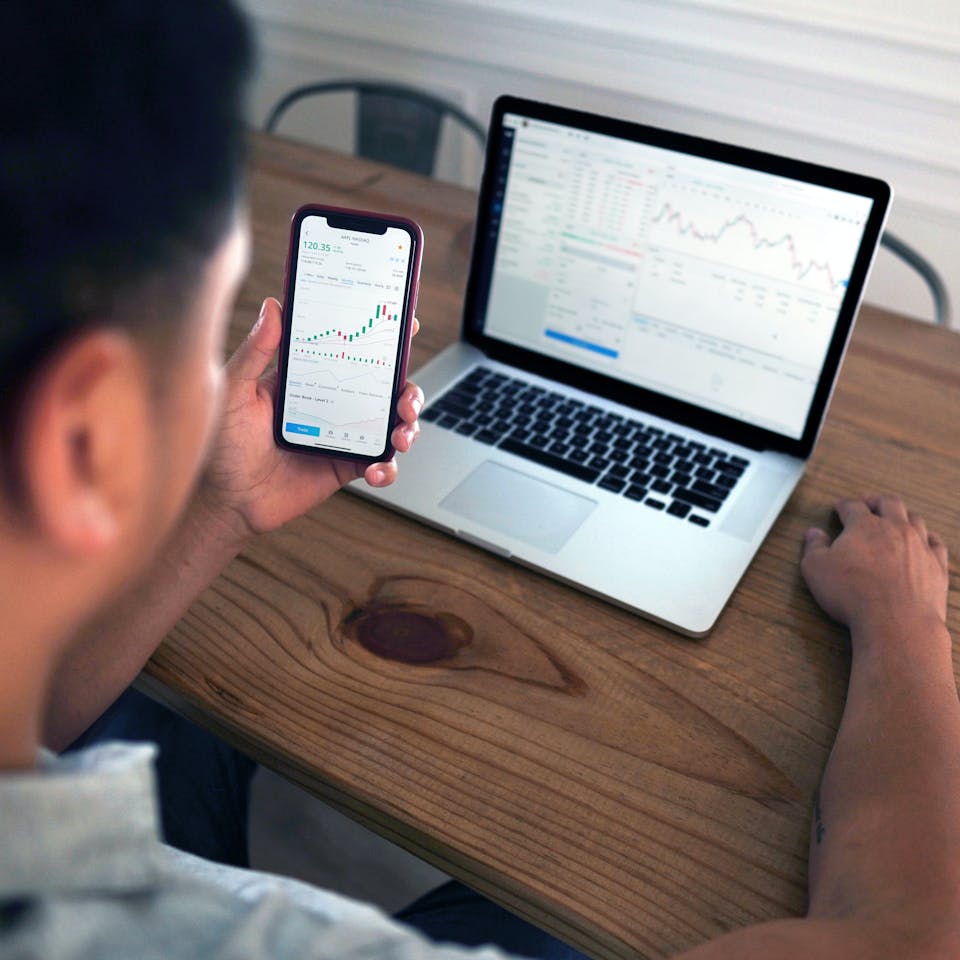

RESEARCH • EDITORIAL
Researchers at The Open University’s Institute of Educational Technology are working with a consortium of European partners to use innovative digital pedagogies, tools and learning design approaches to progress higher education internationally.
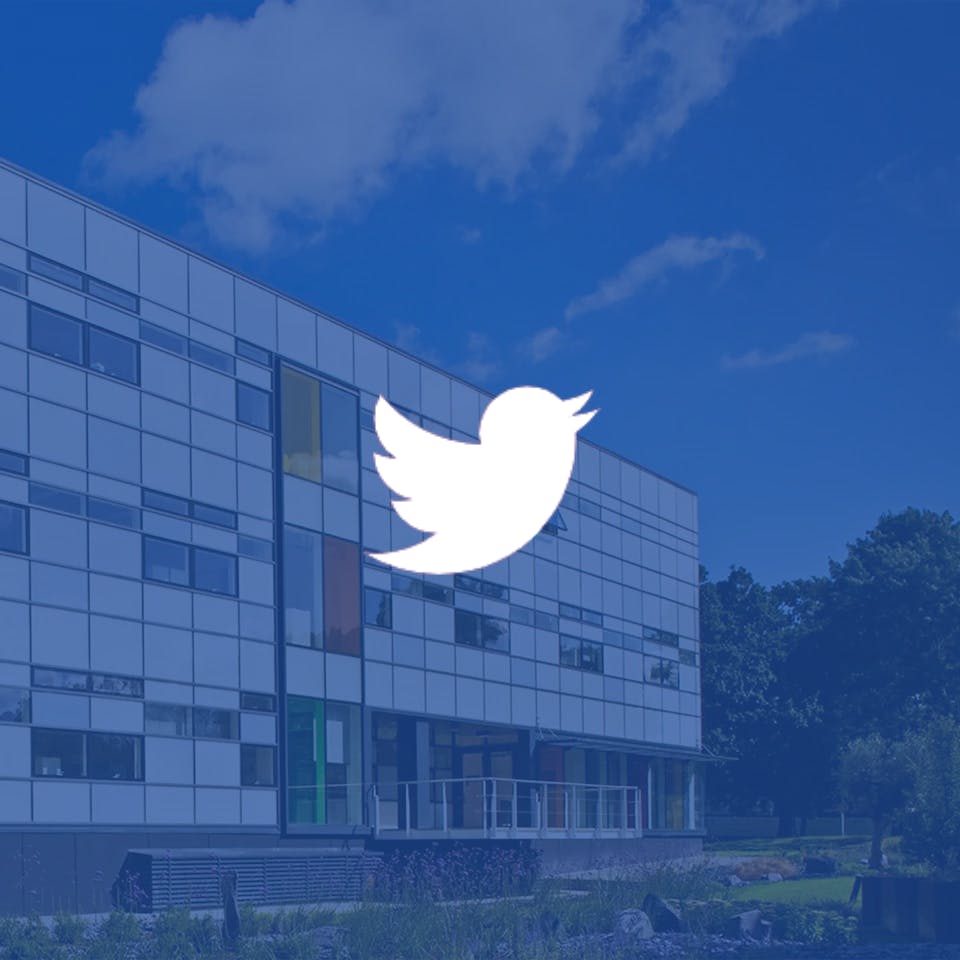

Tweet by @IETatOU •
Professor Christothea Herodotou, Professor Eileen Scanlon and Kevin McLeod from The OU’s Institute of Education Technology (IET) Learning, Teaching and Technologies team are working with partners across Europe, applying expertise in design thinking and harnessing the power of the latest technology, to co-create the next generation of in-person and online teaching. Find out about this #OUResearch here.

STUDY WITH US • EDITORIAL
Dr Lesley Boyd discusses how technology-enabled learning networks can develop internal capabilities for organisational learning, during her doctoral research at The Open University (OU).

RESEARCH • EDITORIAL
Professor Anne Adams, Chair in Engaged Research and Practice at The Open University, has been appointed a UK Parliamentary Office for Science and Technology (POST) Academic Fellowship, developing evidence-based and systematic horizon scanning methodologies, to enable parliamentarians to think one step ahead of the next wave of change.

RESEARCH • EDITORIAL
Research conducted by academic and professional staff at the OU’s Institute of Educational Technology (IET) has been recognised with seven nominations for this year’s Open University Research Excellence Awards.
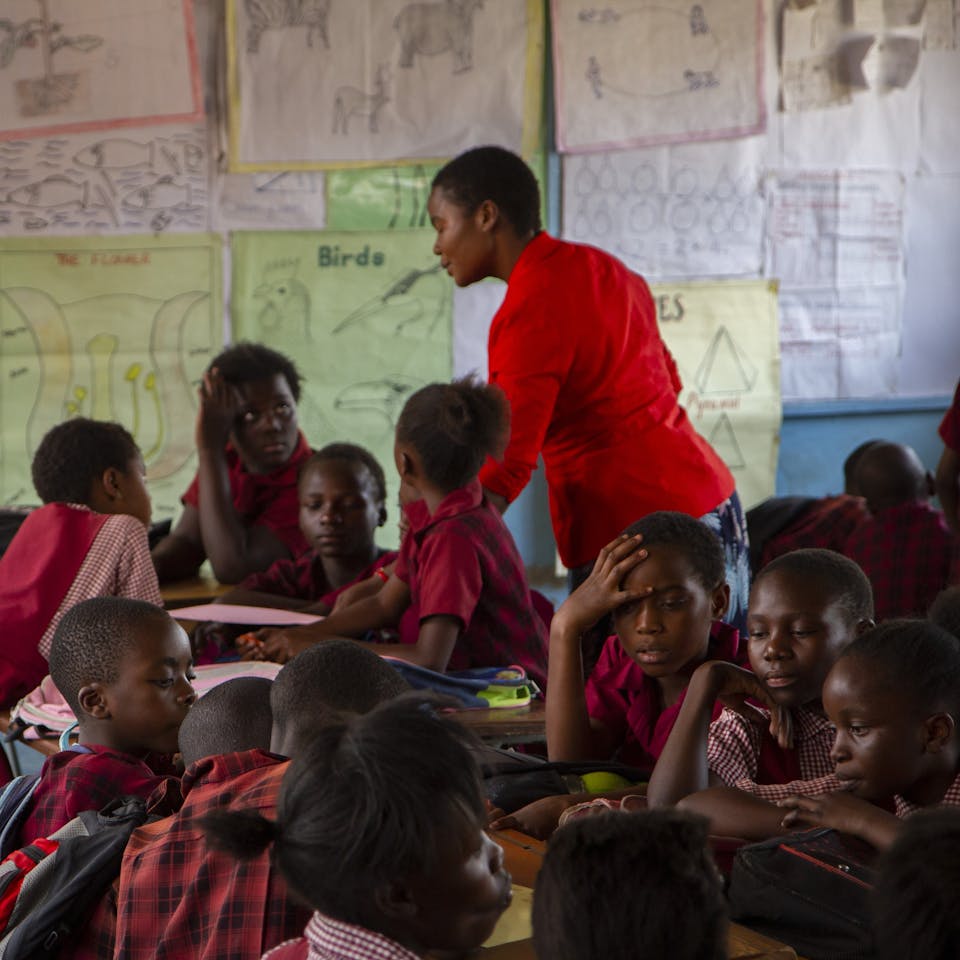

RESEARCH • EDITORIAL
IET is working with the ZEST project, supporting the use of open educational resources and collaborative digital learning approaches.

RESEARCH • EDITORIAL
Through 2020 to 2022, Denise has led various research and knowledge exchange projects across the unit.

RESEARCH • THOUGHT LEADERSHIP
IET are working with Natural History Museums.

RESEARCH • EDITORIAL
The OU and Mental Health Foundation lead citizen science mental health research.

RESEARCH • THOUGHT LEADERSHIP
Research led by IET is exploring how marginalised children, their parents and teachers, use technology to access education. Dr Saraswati Dawadi discusses how effective data collection can uncover lived experiences in under-resourced countries.
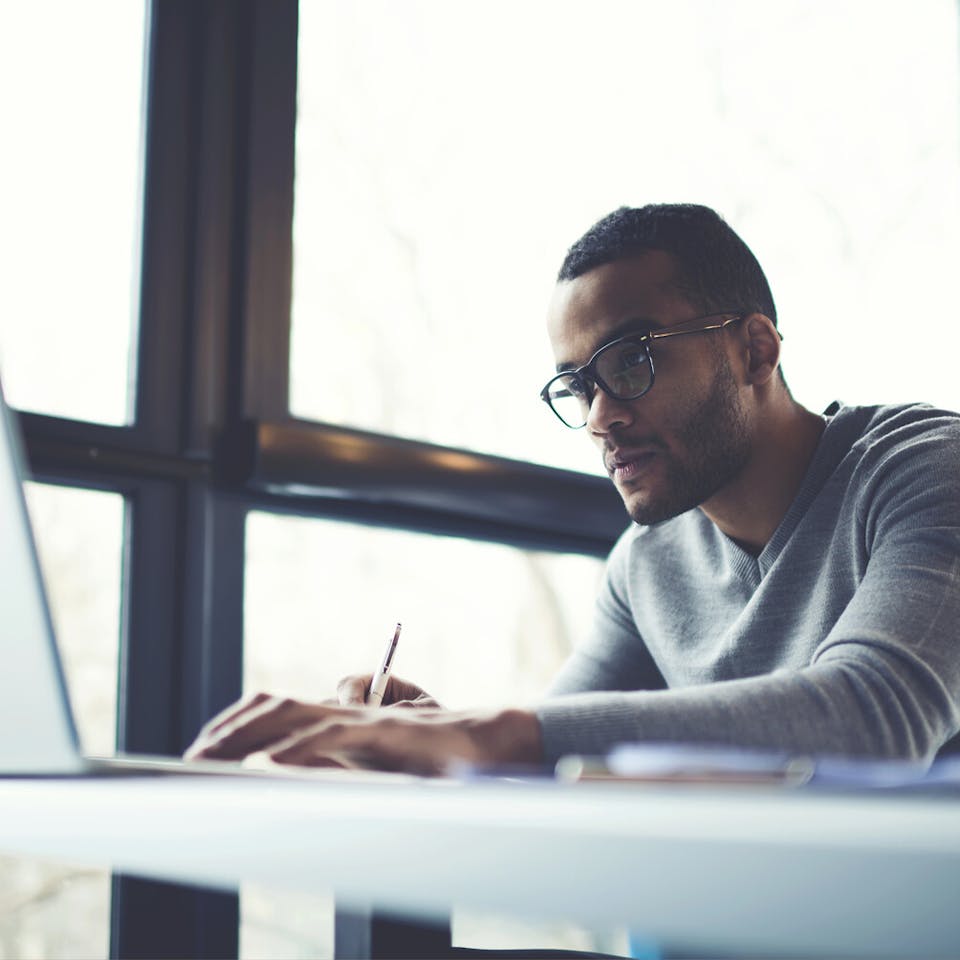

RESEARCH • EDITORIAL
In October 2022, academic researchers from The Open University’s Institute of Educational Technology (IET) were recognised by the awarding panel of the European Conference on e-Learning for their innovative practice on the Skills for Prosperity Kenya programme.
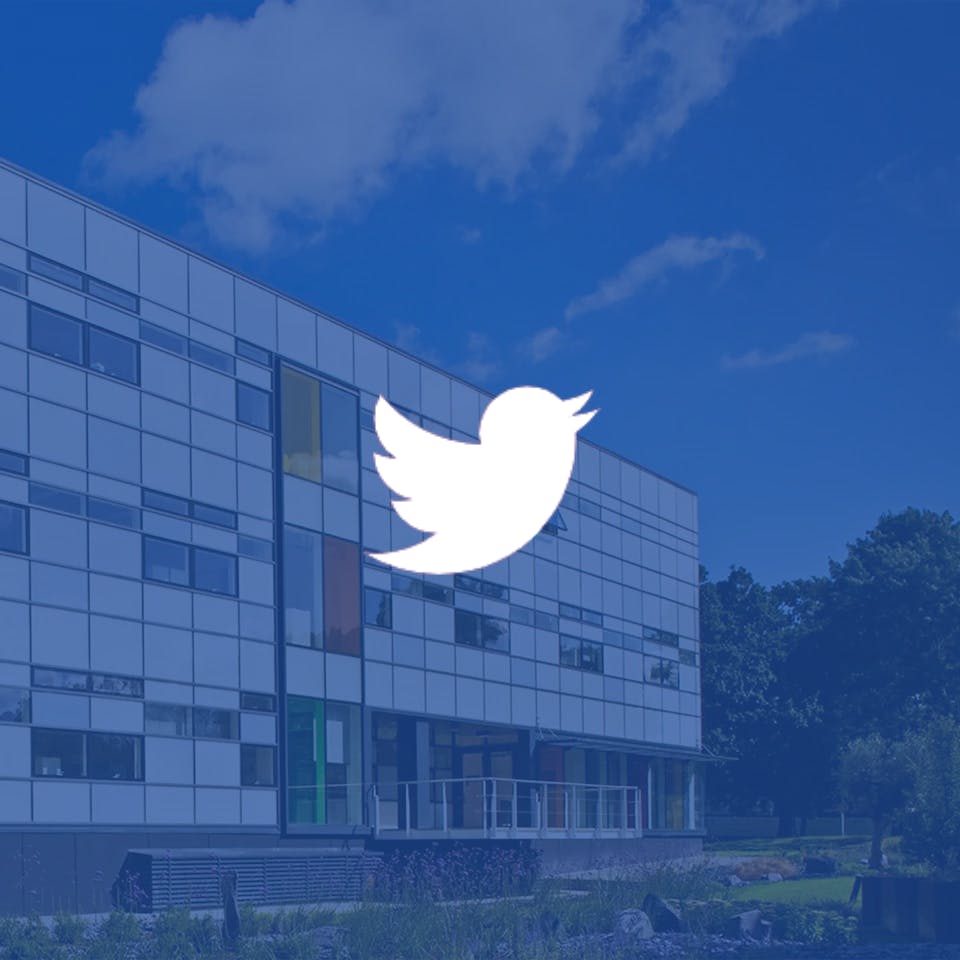

Tweet by @IETatOU •
When Alison began learning online during lockdown, she had no idea how quickly she would be applying her new skills in work. Read how Alison applied practice from the IET-led Online Teaching #microcredential short course in her role based in community learning.

RESEARCH
IET hosts the OU COVID-19 Research: Online Learning and Education seminar.

MICROCREDENTIALS • EDITORIAL
Supporting the design and delivery of accessible and inclusive online courses.

PEOPLE • EDITORIAL
Fereshte joined IET with the aim to engage in research with big impact.
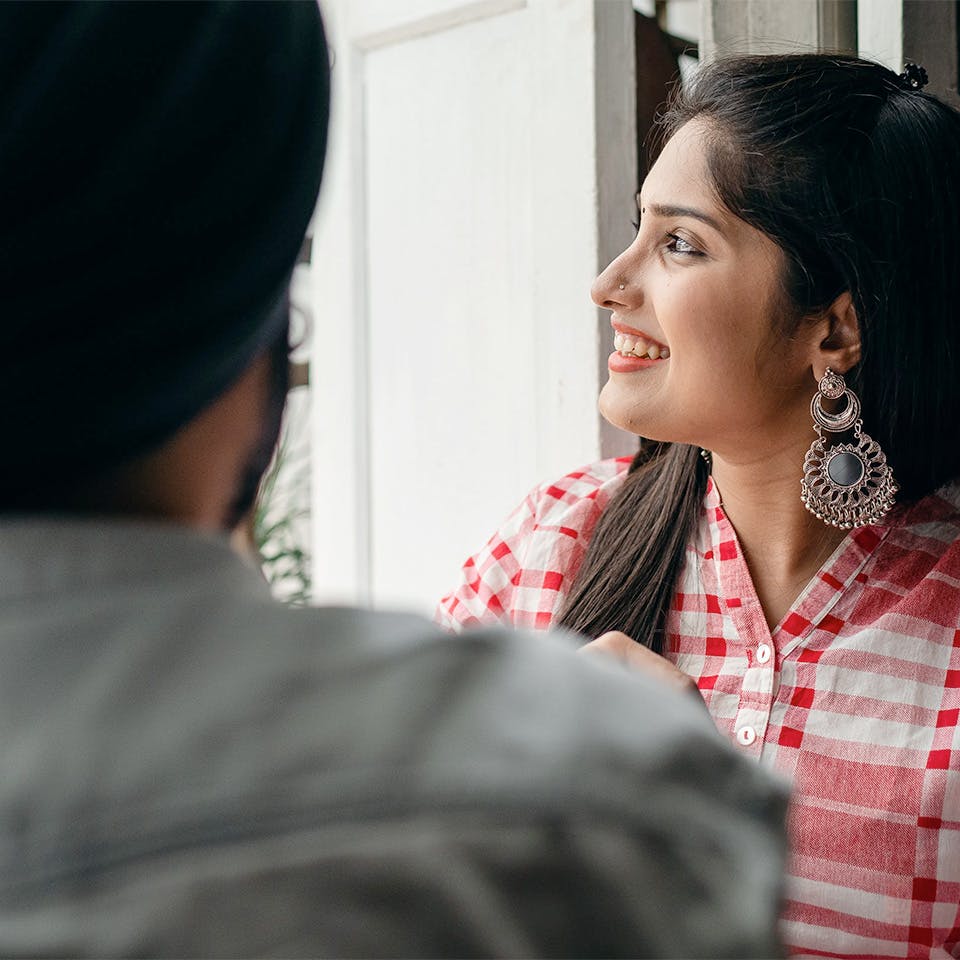

RESEARCH • THOUGHT LEADERSHIP
Digital Badges can support teaching challenges faced during Covid-19.
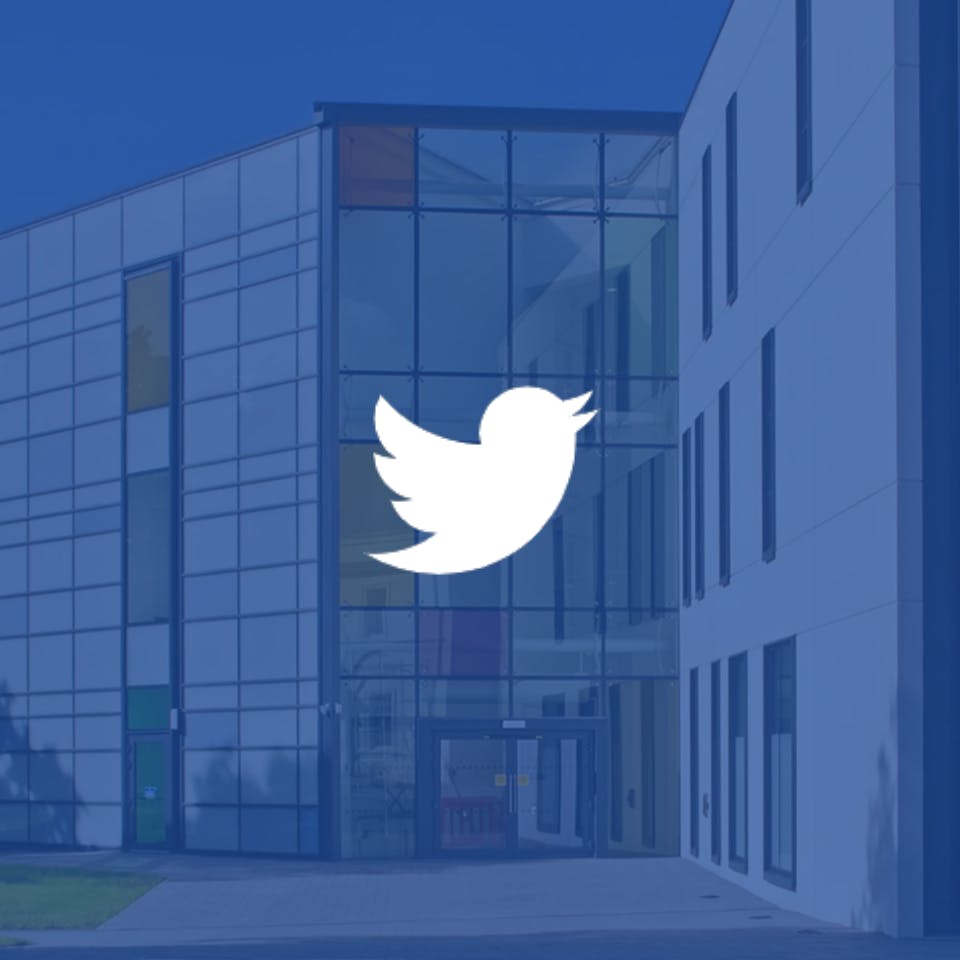

Tweet by @IETatOU •
IET's Dr Leigh-Anne Perryman, senior lecturer at The Open University, shares in a BBC News story how having a study buddy can support productivity. The story discusses a student who livestreams their study-sessions to thousands on TikTok, and has gained notoriety as a source of support for learners during isolation in the pandemic.

RESEARCH • THOUGHT LEADERSHIP
Professor Fridolin Wild discusses why the time is right for learning in the metaverse to be rolled out into the mainstream.

MICROCREDENTIALS • EDITORIAL
IET have launched Teacher Development: Embedding Mental Health in the Curriculum to support educators embed mental health in their curriculum.

RESEARCH • EDITORIAL
Innovative digital technology has the capability to significantly improve the way that people learn from home.